The Rise of Two-Tower Models in Recommender Systems
A deep-dive into the latest technology used to debias ranking models
Recommender systems are among the most ubiquitous Machine Learning applications in the world today. However, the underlying ranking models are plagued by numerous biases that can severely limit the quality of the resulting recommendations. The problem of building unbiased rankers - also known as unbiased learning to rank, ULTR - remains one of the most important research problems within ML and is still far from being solved.
In this post, we’ll take a deep-dive into one particular modeling approach that has relatively recently enabled the industry to control biases very effectively and thus build vastly superior recommender systems: the two-tower model, where one tower learns relevance and another (shallow) tower learns biases.
While two-tower models have probably been used in the industry for several years, the first paper to formally introduce them to the broader ML community was Huawei’s 2019 PAL paper.
PAL (Huawei, 2019) - the OG two-tower model
Huawei’s paper PAL (“position-aware learning to rank”) considers the problem of position bias within the context of the Huawei app store.
Position bias has been observed over and over again in ranking models across the industry. It simply means that users are more likely to click on items that are shown first. This may be because they’re in a hurry, because they blindly trust the ranking algorithm, or other reasons. Here’s a plot demonstrating position bias in Huawei’s data:
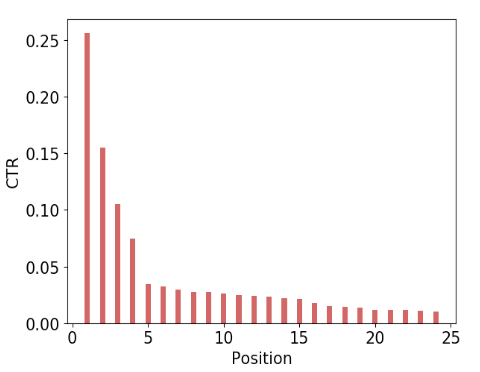
Position bias is a problem because we simply can’t know whether users clicked on the first item because it was indeed the most relevant for them or because it was shown first - and in recommender systems we aim to solve the former learning objective, not the latter.
The solution proposed in the PAL paper is to factorize the learning problem as
p(click|x,position) = p(click|seen,x) x p(seen|position),
where x is the feature vector, and seen
is a binary variable indicating whether the users has seen the impression or not. In PAL, seen
depends only on the position of the item, but we can add other variables as well (as we’ll see later).
Based on this framework, we can then build a model with two towers, each of which outputs one of the two probabilities on the right hand side, and then simply multiply the two probabilities:
The clouds in this image are simply neural networks: a shallow one for the position tower (because it only needs to process a single features), and a deep one for the CTR tower (because it needs to process a large number of features and create interactions between those features). We also call these two towers the bias tower and engagement tower, respectively.
Notably, at inference time, where positions aren’t available, we run a forward pass only using the engagement tower, not the bias tower. Similar to Dropout, the model thus behaves differently at training time at inference time.
Does it work? Yes, indeed, PAL works remarkably well. The authors build 2 different version of the DeepFM ranking model (which I wrote about here), one version with PAL and baseline version with a naive way of treating item position: simply passing it as a feature into the engagement tower. Their online A/B test shows that PAL improves both click-through rates and conversion rates by around 25%, a huge lift!
PAL showed that positions themselves can be used as inputs to a ranking model, but they need to be passed through a dedicated tower, not the main model (a rule that has also been added as Rule 36 to Google’s “Rules of ML”). The two-tower model was officially born - even though it has been likely used across the industry prior to the PAL paper.
Keep reading with a 7-day free trial
Subscribe to Machine Learning Frontiers to keep reading this post and get 7 days of free access to the full post archives.